In the interest of artificial intelligence (AI), deep learning has emerged as a powerful technique that mimics the human brain’s neural networks to solve complex problems. Deep learning algorithms require substantial computational power to train and optimize models effectively. This is where the right hardware comes into play. here you know about the important hardware for deep learning, examining the processors, graphics cards, storage solutions, and other components that can turbocharge your AI capabilities.
Deep learning algorithms are computationally intensive, making it essential to equip your system with the right hardware components. know about the crucial hardware elements that power deep learning projects.
Important Hardware Requirments for Deep Learning
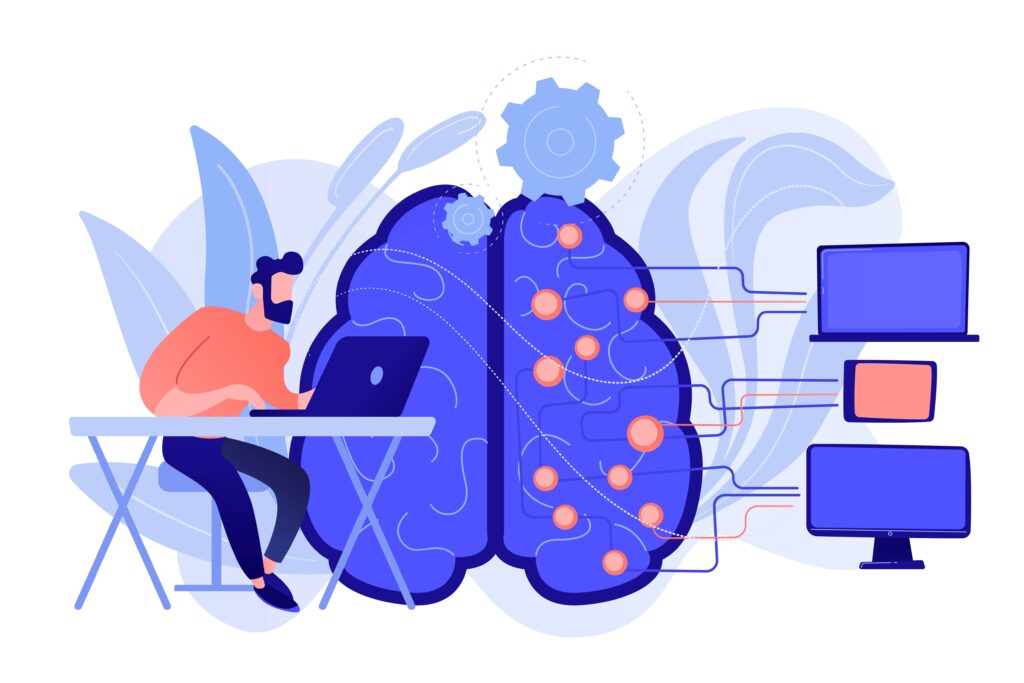
1. High-Performance Processors
Deep learning models heavily rely on the processing power of CPUs (central processing units) to handle the intricate calculations involved in training and inference. To harness the full potential of deep learning, processors with high core counts and excellent multi-threading capabilities are a must. Leading options include.
- AMD Ryzen Threadripper series
- Intel Core i9 processors
- NVIDIA A100 GPUs with Tensor Core technology
These processors deliver the raw power needed to accelerate deep learning tasks, ensuring faster training times and improved model accuracy.
2. Cutting-Edge Graphics Cards
Graphics processing units (GPUs) play a pivotal role in deep learning, as they excel in parallel processing tasks. GPUs are capable of performing numerous calculations simultaneously, making them ideal for training large neural networks. Some of the top GPUs for deep learning applications include.
- NVIDIA GeForce RTX 30 series
- AMD Radeon RX 6000 series
- NVIDIA Quadro RTX series
By harnessing the immense computing power of these GPUs, you can significantly expedite the training process and achieve breakthrough results in your deep learning projects.
3. Ample Memory (RAM)
Deep learning models require substantial memory to store and manipulate large datasets efficiently. Investing in ample RAM capacity ensures smoother operations during training and inference. Recommended memory configurations for deep learning include.
- 32 GB to 64 GB DDR4 RAM for moderate-sized datasets
- 128 GB or more for larger-scale datasets
By providing sufficient memory, you enable your system to handle complex operations without compromising performance.
4. Lightning-Fast Storage Solutions
The speed and responsiveness of storage solutions significantly impact deep learning workflows, especially when dealing with massive datasets. Opt for high-performance storage options like.
- NVMe (Non-Volatile Memory Express) SSDs
- PCIe (Peripheral Component Interconnect Express) SSDs
These cutting-edge storage solutions offer lightning-fast data access, reducing load times and ensuring seamless data processing during training and inference.
5. Reliable Motherboards
To build a robust deep learning system, it’s crucial to select a reliable motherboard that supports your chosen processor and provides ample expansion options. Look for motherboards that offer.
- Compatibility with high-end processors
- Sufficient PCIe slots for multiple GPUs
- Support for large RAM capacities
A sturdy and feature-rich motherboard forms the foundation of a high-performance deep-learning rig.
6. Efficient Cooling Systems
Deep learning workloads can generate substantial heat, putting the hardware at risk of thermal throttling. Effective cooling systems are vital to maintaining optimal operating temperatures during intense computational tasks. Consider the following cooling solutions.
- High-performance CPU coolers
- Liquid cooling solutions
- Case fans for improved airflow
By keeping your hardware cool, you ensure consistent performance and prolong the lifespan of your components.
7. Power Supply Units (PSUs) with Ample Wattage
Deep learning systems, with their high-end processors and graphics cards, demand substantial power to operate efficiently. Investing in a robust power supply unit with sufficient wattage ensures stable and reliable power delivery to all components. Look for PSUs with a minimum rating of 80 Plus Gold for enhanced energy efficiency.
8. Accelerated Computing Platforms
To further accelerate deep learning tasks, consider leveraging specialized accelerated computing platforms. These platforms integrate GPUs and CPUs into a unified architecture, maximizing performance for AI workloads. Notable platforms include.
- NVIDIA CUDA-X AI
- Google Tensor Processing Units (TPUs)
- Amazon EC2 P3 Instances
By utilizing these platforms, you can achieve unparalleled speed and efficiency in your deep learning projects.
9. Peripheral Devices for Enhanced Productivity
While not directly related to deep learning performance, peripheral devices can greatly enhance your productivity and ease of use. Consider investing in.
- High-resolution monitors for better visualization of data and models
- Ergonomic keyboards and mice for comfortable extended usage
- Reliable backup solutions to safeguard valuable datasets and models
These peripheral devices contribute to a seamless deep-learning experience, enabling you to focus on innovation and research.
FAQs about Essential Hardware for Deep Learning
1. Can I use a regular CPU for deep learning tasks?
While it is possible to use a regular CPU for deep learning, high-performance processors specifically designed for AI workloads, such as the AMD Ryzen Threadripper and Intel Core i9 series, deliver significantly better results due to their advanced capabilities.
2. Why are GPUs preferred over CPUs for deep learning?
GPUs excel in parallel processing tasks, allowing them to handle the large-scale matrix calculations required in deep learning with exceptional speed and efficiency. This makes them highly suitable for training complex neural networks.
3. How much RAM do I need for deep learning?
The amount of RAM you need depends on the size of your datasets. For moderate-sized datasets, 32 GB to 64 GB of DDR4 RAM is recommended. Larger-scale datasets may require 128 GB or more to ensure smooth operations.
4. Are NVMe SSDs necessary for deep learning?
While NVMe SSDs offer exceptional speed and performance, they are not essential for deep learning. However, they significantly reduce data loading times and contribute to a smoother workflow, making them highly beneficial for large-scale projects.
5. Can I use multiple GPUs for deep learning?
Yes, using multiple GPUs can significantly accelerate deep learning training times by distributing the workload across multiple devices. However, it requires compatible motherboards with sufficient PCIe slots and appropriate power supply arrangements.
6. Do I need liquid cooling for my deep learning rig?
Liquid cooling is not mandatory for a deep learning rig but can be advantageous, especially when working with high-performance processors and multiple GPUs. It helps dissipate heat more efficiently, ensuring optimal performance during demanding workloads.
Conclusion
In the world of artificial intelligence, deep learning continues to push boundaries and revolutionize various industries. To unlock the true potential of deep learning, it is crucial to equip yourself with the right hardware. From high-performance processors and cutting-edge graphics cards to ample memory, lightning-fast storage solutions, and reliable motherboards, each hardware component plays a vital role in enhancing your deep learning capabilities. By carefully selecting and integrating the essential hardware for deep learning, you can empower your AI projects, achieve faster training times, and unlock groundbreaking insights.
